A Parallel Multi Objective Optimization Genetic Algorithm Gene Feature Selection on Microarray Based Cancer Classification Using Neuro-Fuzzy Inference System [ READ ]
A.Natarajan, Dr.R.Balasubramanian
Feature selection has played a very important role in the field of data mining and machine learning. The high performance parallel and distributed computing is used for gene expression analysis and finding the thousands of genes simultaneously. The classification and validation of molecular biomarkers for cancer diagnosis is an important problem in cancer genomics. The microarray data analysis is used for extracting the biologically useful data from the huge amount of expression data to know the current state of the cell. Most cellular processes are regulated by changes in gene expression. This is a great challenge for computational biologists who see in this new technology the opportunity to discover interactions between genes. In this research we propose a Parallel Multi Objective Optimization Genetic Algorithm for Gene Feature Selection and the best features are evaluated by Adaptive Neuro Fuzzy Inference System classifier. More importantly, the method can exhibit the inherent classification difficulty with respect to different gene expression datasets, indicating the inherent biology of specific cancers
A.Natarajan, Dr.R.Balasubramanian
Feature selection has played a very important role in the field of data mining and machine learning. The high performance parallel and distributed computing is used for gene expression analysis and finding the thousands of genes simultaneously. The classification and validation of molecular biomarkers for cancer diagnosis is an important problem in cancer genomics. The microarray data analysis is used for extracting the biologically useful data from the huge amount of expression data to know the current state of the cell. Most cellular processes are regulated by changes in gene expression. This is a great challenge for computational biologists who see in this new technology the opportunity to discover interactions between genes. In this research we propose a Parallel Multi Objective Optimization Genetic Algorithm for Gene Feature Selection and the best features are evaluated by Adaptive Neuro Fuzzy Inference System classifier. More importantly, the method can exhibit the inherent classification difficulty with respect to different gene expression datasets, indicating the inherent biology of specific cancers
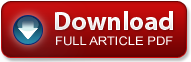